Linear Padding may improve all-in-one metrics ‘stability’.
Writer: Ransourui.
All-in-one metrics’, for example PIPM (player impact plus-minus) and BPM (box plus-minus) etc., ’stability’ is important for decision who will acquire next season. ‘Stability’ means if data are short, the ratings are not calculated extreme values or between season correlation is high.
For stabilize all-in-one metrics, ‘Padding’ methods are used recently. Padding methods add average stats to raw stats. All-in-one metrics are calculated these stats, so padded stats make all-in-one metrics stable.
Padding methods are used for stabilizing all-in-one metrics or stats. Why are all-in-one metrics or stats not stable? My hypothesis is statistical phenomenon called ‘regress to mean’.
‘Regress to mean’ implies extreme good stats will down to mean and extreme bad stats will up to mean.
So, I propose the method, Linear Padding, adjusts ‘regress to mean’ for stabilize all-in-one metrics in this article. And I demonstrate effect of linear padding using Japan Professional Basketball League B League data and EFF (efficiency).
Linear Padding
Linear padding is implemented using linear regression model. For details, first, I modeled relationship between this season stats and next season stat by linear regression model. Second, using the model, I get predicted next season stat. Predicted values are results ‘regress to mean’, so using predicted value for all-in-one metrics correct ‘regress to mean’ effect.
Experiment
I use Japan professional basketball league, B League 2020-21 season and 2021-22 season boxscore data for build regression models. Then, I predict 2022-23 season stats using regression models. Finally, I calculate EFF. I describe how calculate EFF in this article.
EFF=pts + ast + trb + blk + stl – (missed fta+ missed fta +tov).
Each stats are per 36minutes.
I compare correlation coefficients and RMSE between raw EFF vs. 2022-23 season EFF and linear padded EFF vs, 2022-23 EFF. Raw EFF and linear padded EFF are calculated only 2020-21 or 2021-22 season data. If linear padding ‘stabilize’ EFF, correlation coefficient between linear padded EFF and 2022-23 season EFF are higher than correlation coefficient raw EFF and 2022-23 EFF. Moreover, RMSE between linear padded EFF and 2022-23 season EFF are smaller than RMSE between raw EFF and 2022-23 EFF.
Results
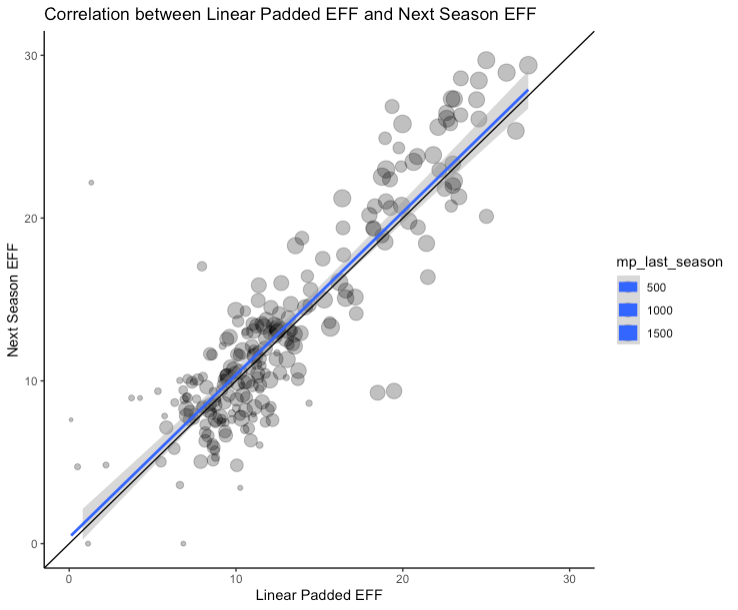
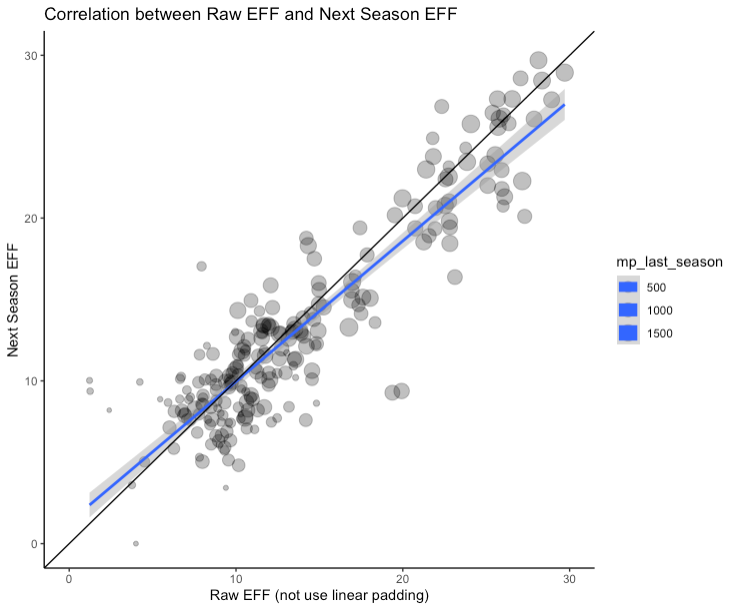
Correlation between linear padded EFF and 2022-23 season EFF is 0.869 and raw EFF and 2022-23 season EFF is 0.854. The difference looks small, but statistically significant (p<0.01)
RMSE between linear padded EFF and 2022-23 season EFF is 2.41 and raw EFF and 2022-23 season EFF is 2.53.
Discussion
Using linear padding, EFF’s between season correlation is higher and RMSE is smaller. I guess linear padding make ‘stabilize’ not only EFF but also other all-in-one metrics.